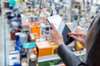
Welcome to the Senseye Predictive Maintenance resources hub
Helping you look beyond single assets and production lines, Senseye Predictive Maintenance is a powerful tool that can fundamentally redefine maintenance processes throughout your organization. Use our comprehensive collection of resources to keep up to date with the latest industry best practices and updates.